Academia Member
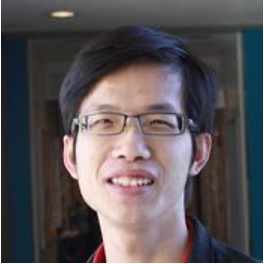
Dr. Edwin Khoo
Physics-based modeling of electrochemical systems e.g. batteries
I am broadly interested in building physics-based models for electrochemical systems such as batteries, supercapacitors and fuel cells that accurately and quantitatively describe dominant physics and chemistries occurring. Such models will enable us to more fundamentally understand how to optimize these complex devices to satisfy key performance metrics such as energy density, power density and cycle life. One particular area of interest is developing multiscale and multiphysics battery models that couple electrochemistry with solid mechanics. These high-fidelity computational models are critical for advancing our understanding of next-generation silicon anodes and solid electrolytes that are needed for realizing safe and high-energy-density batteries.
My PhD research focused on theoretical and numerical analysis, such as linear stability analysis and electrochemical impedance spectrum analysis, of electrodeposition in charged porous media in the context of discovering and exploring new physical mechanisms for enabling high-energy-density metal batteries such as lithium metal batteries. I also contributed to the formulation and verification of a phase field model for describing intercalation dynamics and phase separation in layered materials such as graphite. I am interested in building on my PhD work and applying these techniques to other electrochemical systems such as redox flow batteries and fuel cells.
Machine learning and deep learning
I am interested in using machine learning and deep learning to overcome bottlenecks in the scientific discovery of chemicals and materials, especially for electrochemical energy conversion and storage technologies. Some machine learning and deep learning techniques that are relevant for accelerating scientific discovery include kernel-based models, e.g. Gaussian processes and support vector machines, and neural-network-based models, e.g. feedforward, convolutional and recurrent neural networks. These techniques can be leveraged for the closed loop design of chemicals and materials in which high-throughput automated experimentation is coupled with data-driven exploration of the chemical and material spaces.
I am particularly interested in using machine learning to accelerate translation of laboratory-scale discoveries to industrial-scale manufacturing because this translation is historically a critical bottleneck in commercializing promising laboratory-scale battery results. Manufacturability and optimization of processing conditions and materials choices are key issues to be considered and addressed under this framework.
Integration of data-driven machine learning approaches with physics-based models
Modern data-driven machine learning and deep learning models excel at fitting high-dimensional complex data using sufficiently large datasets. However, they are often not easily interpretable and explainable, and datasets in many domains including batteries are usually not large enough for cutting-edge deep learning models. On the other hand, physics-based models are easily interpretable and explainable but may lack sufficient accuracy because of incomplete model specification. Therefore, I am interested in integrating physics-based models with machine learning approaches in order to tap into their respective strengths and maximize accuracy, interpretability and explainability.